Price Testing for Mobile Apps
An end-to-end solution for running price experiments.
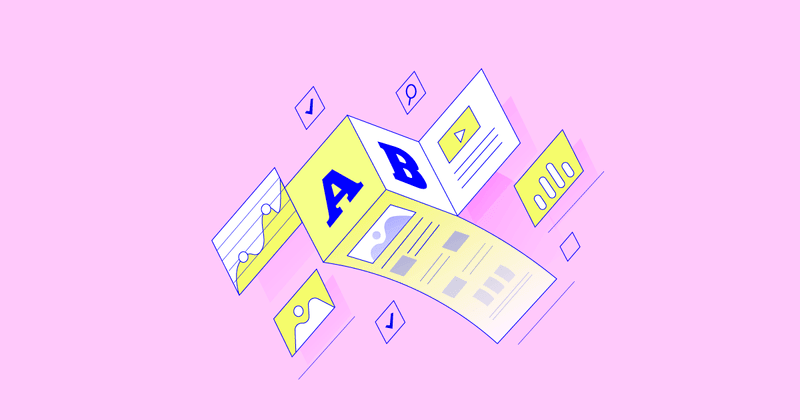
A/B price testing for mobile apps is hard — to get it right, you have to effectively manage user cohorting, data collection, and data analysis. Add in the complexity introduced by subscriptions, and it quickly becomes a problem that most developers won’t tackle — or worse, do incorrectly.
Our mission at RevenueCat is to help developers make more money, and pricing is one of the most effective levers for increasing revenue. While price experiments are difficult to do well, they’re the best way to measure the impact of pricing changes.
We love making hard things easy for our users, so we tackled this problem head-on. To that end, we’re excited to share Experiments, our take on A/B testing in mobile subscription app businesses and the easiest way to run high-tempo, high-fidelity pricing tests in your app.
The math of A/B tests
A typical A/B test goes something like this: Split users randomly into two cohorts, show each cohort a different version of the app, then measure how many users from each group performed some action. This action might be clicking a button, signing up, or sharing to Twitter; most A/B significance calculators you find online assume a situation along these lines. In statistics land, we call this a binomial experiment — a trial is either successful or not. In this case, success is defined as taking the desired action.

The math is a little complex, but after running a binomial experiment, you can then determine whether the measured success rate for one group is higher than that of the other as well as the likelihood that it’s more than just a statistical fluke. This likelihood is called significance and is at the heart of why experiments are difficult to do well.
Measuring success in price experiments
A binomial experiment is a great way to test many areas of your app. However, not all measures of success are as simple as assessing whether or not a user takes an action.
Price experiments are a great example of this. Measuring how many users purchase something is binomial — they either purchase it or they don’t) — but what about when the price changes? In this case, the actions of each cohort are different; a lower price may equal more purchases but not necessarily more revenue, and it’s not possible to measure the impact of the change accurately via a simple “yes/no” purchase decision metric. Add in the complexity of the users’ subscriptions — did they start a trial, did they convert to a paid plan, and how many times did they renew? — and measuring success becomes very complicated very quickly.
The nuances of app subscriptions make price experiments extremely difficult to do well without understanding how your change impacted the full subscription lifecycle of your customer journey. To solve this, we’ve built an end-to-end view of the subscription lifecycle to make it easy to see and understand the full impact of your price experiment.
The subscription lifecycle
The goal of any price experiment is to understand which price point yields the highest lifetime value (LTV) per customer: the revenue you generate across some time horizon. That time horizon is usually specific to each business: You might measure your lifetime value based on a one-year, three-year, or unbounded lifetime, but the common denominator is the same: It’s an extended period.
This is the blessing and curse of subscription businesses. As you deliver value consistently to your subscribers, they deliver value back to you in the form of renewals. However, that means the value you’ll derive from each subscriber is unknowable on the first day of the subscription.
Introducing RevenueCat Experiments
We built Experiments to help you easily run, analyze, and draw conclusions from a/b price testing for mobile apps with the full subscription lifecycle in mind.
If you’re using RevenueCat Offerings to configure which products you offer in your app, you’re already set up to use Experiments through RevenueCat. Just set up the two offerings you want to test against each other, turn on your experiment, and we’ll start splitting customers evenly between those two variants (more details here).
How we approach price experiment results
Remember: Lifetime value is the key metric you’re looking to grow through your price experiment. Accordingly, when analyzing the impact of your price experiments on LTV you’ll ultimately generate per customer, it’s helpful to group the metrics of the subscription lifecycle into three buckets:
- Initial conversion: This is the point where your customer starts a free trial (or accepts some other offer) and where you’ll see the most immediate impact from your experimentation.
- Paid customers: For most subscription businesses, this will be the key crossover point where a customer becomes a revenue-generating subscriber who contributes to your lifetime value.
- Revenue: You can think of the revenue you’ve generated during an experiment as the “Realized LTV” of those customer cohorts. However, keep in mind that LTV will grow over time as you retain active subscribers from those cohorts; the current value is an imperfect snapshot of the full impact of price changes over time. The rate of growth may also differ based on your price experiment and the composition of your paid customers (e.g., the percent of annual subscribers vs. monthly).
For each group, RevenueCat offers a handful of specific metrics to analyze in order to ensure that you have a clear picture of what’s driving performance changes in your price experiment:
Initial conversion | Paid customers | Revenue |
Initial conversions | Paid customers | Realized LTV (revenue) |
Initial conversion rate | Conversion to paying | Realized LTV per customer |
Trials started | Active subscribers | Realized LTV per paying customer |
Trials completed | Churned subscribers | Total MRR |
Trials converted | Refunded customers | MRR per customer |
Trial conversion rate | MRR per paying customer |
A snapshot of your results over time
When you run a price experiment in RevenueCat, we’ll show you how each metric within the customer journey has changed over time, so you can quickly see which trends are consistent, which ones are volatile, and where to dig deeper.
You can also easily filter these results by platform to see how performance differs between them, and you can export the full history of each metric by day for additional analysis.
Compare the full customer journey
To see the performance of specific metrics side by side and dig deeper into the change drivers, you’ll also see tables of the cumulative performance of each metric in the subscription lifecycle for each variant.
In addition, you can expand most metrics to view performance by product to understand exactly where performance changes are coming from. Of course, you can also export this tabular data for your own deeper investigation.
You can’t make a high-confidence decision on a price experiment without deeply understanding what’s driving the changes you’re observing. We think this comprehensive view of the subscription lifecycle will give you the visibility you need to know what’s changed and why so you can make the right decision for your product and drive customer LTV through optimized pricing.
Run your next price experiment with RevenueCat
We’re excited to launch RevenueCat Experiments to all our Pro and Enterprise developers. Ready to try it? You can get started with Experiments here.
RevenueCat’s app monetization platform empowers developers to confidently deploy in-app purchases and subscriptions, centralize data, and grow revenue across iOS, Android, and the web. Explore implementing RevenueCat for your app to gain access to tools Experiments, Charts, Integrations and more.
You might also like
- Blog post
Navigating Google Play’s 14-Day testing rule: a Shipaton survival guide
This article explains Google Play’s 14-day testing rule and how to start the clock immediately to ensure it doesn’t delay your submission.
- Blog post
A beginner’s guide to implementing ad-free subscriptions in your React Native app
A step-by-step tutorial to let users pay to remove ads—using Expo, AdMob, and RevenueCat
- Blog post
How to build a Blinkist-style paywall using RevenueCat webhooks and Zapier
Build a Blinkist-style paywall with RevenueCat and Zapier—no backend required.